Members of ACCURE Battery Intelligence have been spearheading the development of battery diagnostic solutions for over a decade. Our journey began as scientists within a research project at RWTH Aachen University, involving 21 private households using first generation home storage systems. From there, we’ve continuously refined our battery diagnostic algorithms and tailored them to industry needs.
Why This Research Matters
Home storage systems are a pivotal component in driving the decentralized energy transition, and more so – empowering individuals to take part in it. The global market has grown significantly in recent years, with Germany alone being home to 1.5 million installed systems. As the European Batteries Regulation now requires reliable estimates of battery health (typically measured by remaining capacity), this research couldn’t be timelier.
How Home Storage Systems Work
Home storage systems are ingeniously simple: they charge with surplus photovoltaic (PV) energy during the day and supply this energy to households at night.
While some systems charge as soon as there is surplus PV power (excess-charging strategy), others wait until noon to charge, targeting the PV generation peak (forecast-based strategy). The forecast-based strategy is advantageous for two reasons:
- Maximizing PV generation: By reducing the maximum PV feed-in power due to the charge at noon, more PV energy can be generated in total as no curtailment occurs.
- Reducing battery aging: Batteries age fast at high SOCs. A delayed charge reduces battery aging because the average SOC is kept lower than systems under the excess-charging strategy.
Figure 1 below shows examples of these two two operational strategy types. The excess-charging system on the left begins charging (positive power) early in the morning, causing it to reach a fully charged state around noon. As a result, it must curtail PV power during midday to comply with the 60% feed-in limit of the subsidy program in this case. In contrast, the forecast-based system on the right delays charging until noon, allowing it to fully utilize the peak PV generation.

Figure 1: Excess-charging (left) vs. forecast-based (right) operational strategy. Figure according to Figgener et al. (2024), https://www.nature.com/articles/s41560-024-01620-9
How to Estimate Solar Battery Capacity
Home storage systems are an interesting application for battery diagnostics. Their regular full charge and discharge cycles make it possible to precisely determine capacity. Because of this operational behavior, our method tailors coulomb counting to the specifics of home storage operation:
- Determining end-of-charge and end-of-discharge voltages
- Integrating current between these voltages
- Accounting for offset current to accurately determine capacity
As shown in Figure 2, the resulting algorithmic state of health (SOH) estimation (blue dots) closely matches the conducted field capacity tests (yellow dots). Using a simplified linear fit (illustrated by the red trendline in Figure 2), the system’s aging rate is determined to be 2.7 percentage points per year. Based on this rate, the system is estimated to reach its end of life of 80% SOH in about 7 years, which coincides with the warranty period given at the time.
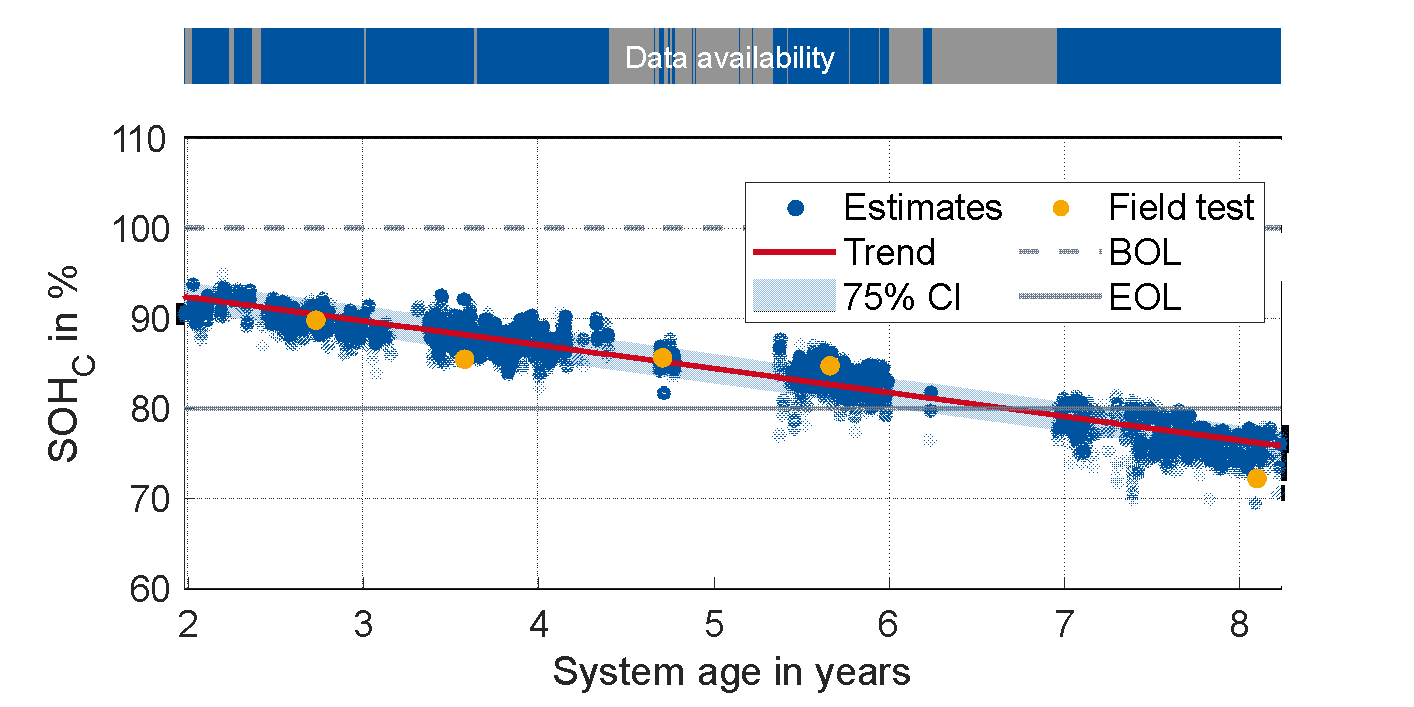
Figure 2: State of health (SOH) estimation. Algorithmic capacity estimation (blue dots) vs. field capacity tests (yellow dots). Field measurements for the system began two years after commissioning (see start of x-axis). Figure according to Figgener et al. (2024), https://www.nature.com/articles/s41560-024-01620-9
Our method is validated for lithium nickel manganese cobalt oxide (NMC), lithium nickel manganese oxide (LMO), and lithium iron phosphate (LFP) batteries, delivering robust results across different systems. Overall, we found that home storage systems lose 2-3 percentage points of usable capacity per year. On a positive note, most first-generation products met their warranties by adding a capacity buffer.